Most Frequently Asked Artificial Intelligence Interview Questions
Artificial Intelligence Basic Level Interview Questions
Q1. What is Artificial Intelligence? Give an example of where AI is used on a daily basis.
“Artificial Intelligence (AI) is an area of computer science that emphasizes the creation of intelligent machines that work and react like humans.” “The capability of a machine to the intelligent imitate human behavior.”
Google’s Search Engine – Artificial Intelligence Interview Questions
Google’s Search Engine
One of the most popular AI Applications is the google search engine. If you open up your chrome browser and start typing something, Google immediately provides recommendations for you to choose from. The logic behind the search engine is Artificial Intelligence.
AI uses predictive analytics, NLP and Machine Learning to recommend relevant searches to you. These recommendations are based on data that Google collects about you, such as your search history, location, age, etc. Thus, Google makes use of AI, to predict what you might be looking for.
Q2. What are the different types of AI?
- Reactive Machines AI: Based on present actions, it cannot use previous experiences to form current decisions and simultaneously update their memory.
Example: Deep Blue - Limited Memory AI: Used in self-driving cars. They detect the movement of vehicles around them constantly and add it to their memory.
- Theory of Mind AI: Advanced AI that has the ability to understand emotions, people and other things in the real world.
- Self Aware AI: AIs that posses human-like consciousness and reactions. Such machines have the ability to form self-driven actions.
- Artificial Narrow Intelligence (ANI): General purpose AI, used in building virtual assistants like Siri.
- Artificial General Intelligence (AGI): Also known as strong AI. An example is the Pillo robot that answers questions related to health.
- Artificial Superhuman Intelligence (ASI): AI that possesses the ability to do everything that a human can do and more. An example is the Alpha 2 which is the first humanoid ASI robot.
Q3. Explain the different domains of Artificial Intelligence.
- Machine Learning: It’s the science of getting computers to act by feeding them data so that they can learn a few tricks on their own, without being explicitly programmed to do so.
- Neural Networks: They are a set of algorithms and techniques, modeled in accordance with the human brain. Neural Networks are designed to solve complex and advanced machine learning problems.
- Robotics: Robotics is a subset of AI, which includes different branches and application of robots. These Robots are artificial agents acting in a real-world environment. An AI Robot works by manipulating the objects in it’s surrounding, by perceiving, moving and taking relevant actions.
- Expert Systems: An expert system is a computer system that mimics the decision-making ability of a human. It is a computer program that uses artificial intelligence (AI) technologies to simulate the judgment and behavior of a human or an organization that has expert knowledge and experience in a particular field.
- Fuzzy Logic Systems: Fuzzy logic is an approach to computing based on “degrees of truth” rather than the usual “true or false” (1 or 0) boolean logic on which the modern computer is based. Fuzzy logic Systems can take imprecise, distorted, noisy input information.
- Natural Language Processing: Natural Language Processing (NLP) refers to the Artificial Intelligence method that analyses natural human language to derive useful insights in order to solve problems.
Q4.Explain the commonly used Artificial Neural Networks.
Feedforward Neural Network
- The simplest form of ANN, where the data or the input travels in one direction.
- The data passes through the input nodes and exit on the output nodes. This neural network may or may not have the hidden layers.
Convolutional Neural Network
- Here, input features are taken in batch wise like a filter. This will help the network to remember the images in parts and can compute the operations.
- Mainly used for signal and image processing
Recurrent Neural Network(RNN) – Long Short Term Memory
- Works on the principle of saving the output of a layer and feeding this back to the input to help in predicting the outcome of the layer.
- Here, you let the neural network to work on the front propagation and remember what information it needs for later use
- This way each neuron will remember some information it had in the previous time-step.
Autoencoders
- These are unsupervised learning models with an input layer, an output layer and one or more hidden layers connecting them.
- The output layer has the same number of units as the input layer. Its purpose is to reconstruct its own inputs.
- Typically for the purpose of dimensionality reduction and for learning generative models of data.
Q5. How is Machine Learning related to Artificial Intelligence?
Artificial Intelligence is a technique that enables machines to mimic human behavior. Whereas, Machine Learning is a subset of Artificial Intelligence. It is the science of getting computers to act by feeding them data and letting them learn a few tricks on their own, without being explicitly programmed to do so. Therefore Machine Learning is a technique used to implement Artificial Intelligence.
Q6. What is Deep Learning?
Deep learning imitates the way our brain works i.e. it learns from experiences. It uses the concepts of neural networks to solve complex problems.
Any Deep neural network will consist of three types of layers:
- Input Layer: This layer receives all the inputs and forwards them to the hidden layer for analysis
- Hidden Layer: In this layer, various computations are carried out and the result is transferred to the output layer. There can be n number of hidden layers, depending on the problem you’re trying to solve.
- Output Layer: This layer is responsible for transferring information from the neural network to the outside world.
Q7. Explain how Deep Learning works.
- Deep Learning is based on the basic unit of a brain called a brain cell or a neuron. Inspired from a neuron, an artificial neuron or a perceptron was developed.
- A biological neuron has dendrites which are used to receive inputs.
- Similarly, a perceptron receives multiple inputs, applies various transformations and functions and provides an output.
- Just like how our brain contains multiple connected neurons called neural network, we can also have a network of artificial neurons called perceptron’s to form a Deep neural network.
An Artificial Neuron or a Perceptron models a neuron which has a set of inputs, each of which is assigned some specific weight. The neuron then computes some function on these weighted inputs and gives the output.
Q8. What are Bayesian Networks?
A Bayesian network is a statistical model that represents a set of variables and their conditional dependencies in the form of a directed acyclic graph.
On the occurrence of an event, Bayesian Networks can be used to predict the likelihood that any one of several possible known causes was the contributing factor.
For example, a Bayesian network could be used to study the relationship between diseases and symptoms. Given various symptoms, the Bayesian network is ideal for computing the probabilities of the presence of various diseases.
Q9. What is Q-Learning?
The Q-learning is a Reinforcement Learning algorithm in which an agent tries to learn the optimal policy from its past experiences with the environment. The past experiences of an agent are a sequence of state-action-rewards:
Artificial Intelligence Intermediate Level Interview Questions
Q1. How is Computer Vision and AI related?
Computer Vision is a field of Artificial Intelligence that is used to obtain information from images or multi-dimensional data. Machine Learning algorithms such as K-means is used for Image Segmentation, Support Vector Machine is used for Image Classification and so on.
Therefore Computer Vision makes use of AI technologies to solve complex problems such as Object Detection, Image Processing, etc.
Q2. What is the purpose of Deep Learning frameworks such as Keras, TensorFlow, and PyTorch?
- Keras is an open source neural network library written in Python. It is designed to enable fast experimentation with deep neural networks.
- TensorFlow is an open-source software library for dataflow programming. It is used for machine learning applications like neural networks.
- PyTorch is an open source machine learning library for Python, based on Torch. It is used for applications such as natural language processing.
Q3. How does Reinforcement Learning work? Explain with an example.
Generally, a Reinforcement Learning (RL) system is comprised of two main components:
- An agent
- An environment
- The environment is the setting that the agent is acting on and the agent represents the RL algorithm.
- The RL process starts when the environment sends a state to the agent, which then based on its observations, takes an action in response to that state.
- In turn, the environment sends the next state and the respective reward back to the agent. The agent will update its knowledge with the reward returned by the environment to evaluate its last action.
- The loop continues until the environment sends a terminal state, which means the agent has accomplished all his tasks.
To understand this better, let’s suppose that our agent is learning to play counter strike. The RL process can be broken down into the below steps:
- The RL Agent (Player1) collects state S⁰ from the environment (Counterstrike game)
- Based on the state S⁰, the RL agent takes an action A⁰, (Action can be anything that causes a result i.e. if the agent moves left or right in the game). Initially, the action is random
- The environment is now in a new state S¹ (new stage in the game)
- The RL agent now gets a reward R¹ from the environment. This reward can be additional points or coins
- This RL loop goes on until the RL agent is dead or reaches the destination, and it continuously outputs a sequence of state, action, and reward.
To learn more about Reinforcement Learning you can go through this video recorded by our Machine Learning experts.
Q4. Explain reward maximization in Reinforcement Learning.
The RL agent works based on the theory of reward maximization. This is exactly why the RL agent must be trained in such a way that, he takes the best action so that the reward is maximum.
Let me explain this with a small game. In the figure you can see a fox, some meat and a tiger.
- Our RL agent is the fox and his end goal is to eat the maximum amount of meat before being eaten by the tiger.
- Since this fox is a clever fellow, he eats the meat that is closer to him, rather than the meat which is close to the tiger, because the closer he is to the tiger, the higher are his chances of getting killed.
- As a result, the rewards near the tiger, even if they are bigger meat chunks, will be discounted. This is done because of the uncertainty factor, that the tiger might kill the fox.
The next thing to understand is, how discounting of rewards work?
To do this, we define a discount rate called gamma. The value of gamma is between 0 and 1. The smaller the gamma, the larger the discount and vice versa.
Q5. What is exploitation and exploration trade-off?
An important concept in reinforcement learning is the exploration and exploitation trade-off.
Exploration, like the name suggests, is about exploring and capturing more information about an environment. On the other hand, exploitation is about using the already known exploited information to heighten the rewards.
Exploitation & Exploration – Artificial Intelligence Interview Questions
Consider the fox and tiger example, where the fox eats only the meat (small) chunks close to him but he doesn’t eat the bigger meat chunks at the top, even though the bigger meat chunks would get him more rewards.
- If the fox only focuses on the closest reward, he will never reach the big chunks of meat, this is called exploitation.
- But if the fox decides to explore a bit, it can find the bigger reward i.e. the big chunk of meat. This is exploration.
Q6. What are hyperparameters in Deep Neural Networks?
- Hyperparameters are variables that define the structure of the network. For example, variables such as the learning rate, define how the network is trained.
- They are used to define the number of hidden layers that must be present in a network.
- More hidden units can increase the accuracy of the network, whereas a lesser number of units may cause underfitting.
Q7. Explain the different algorithms used for hyperparameter optimization.
Grid search trains the network for every combination by using the two set of hyperparameters, learning rate and the number of layers. Then evaluates the model by using Cross Validation techniques.
Random Search
It randomly samples the search space and evaluates sets from a particular probability distribution. For example, instead of checking all 10,000 samples, randomly selected 100 parameters can be checked.
Bayesian Optimization
This includes fine-tuning the hyperparameters by enabling automated model tuning. The model used for approximating the objective function is called surrogate model (Gaussian Process). Bayesian Optimization uses Gaussian Process (GP) function to get posterior functions to make predictions based on prior functions.
Q8. How does data overfitting occur and how can it be fixed?
Overfitting occurs when a statistical model or machine learning algorithm captures the noise of the data. This causes an algorithm to show low bias but high variance in the outcome.
Overfitting can be prevented by using the following methodologies:
Cross-validation: The idea behind cross-validation is to split the training data in order to generate multiple mini train-test splits. These splits can then be used to tune your model.
More training data: Feeding more data to the machine learning model can help in better analysis and classification. However, this does not always work.
Remove features: Many times, the data set contains irrelevant features or predictor variables that are not needed for analysis. Such features only increase the complexity of the model, thus leading to possibilities of data overfitting. Therefore, such redundant variables must be removed.
Early stopping: A machine learning model is trained iteratively, this allows us to check how well each iteration of the model performs. But after a certain number of iterations, the model’s performance starts to saturate. Further training will result in overfitting, thus one must know where to stop the training. This can be achieved by a mechanism called early stopping.
Regularization: Regularization can be done in n number of ways, the method will depend on the type of learner you’re implementing. For example, pruning is performed on decision trees, the dropout technique is used on neural networks and parameter tuning can also be applied to solve overfitting issues.
Use Ensemble models: Ensemble learning is a technique that is used to create multiple Machine Learning models, which are then combined to produce more accurate results. This is one of the best ways to prevent overfitting. An example is Random Forest, it uses an ensemble of decision trees to make more accurate predictions and to avoid overfitting.
Q9. Mention a technique that helps to avoid overfitting in a neural network.
Dropout is a type of regularization technique used to avoid overfitting in a neural network. It is a technique where randomly selected neurons are dropped during training.
The Dropout value of a network must be chosen wisely. A value too low will result in a minimal effect and a value too high results in under-learning by the network.
Q10. What are the different components of NLP?
Natural Language Understanding includes:
- Mapping input to useful representations
- Analyzing different aspects of the language
Natural Language Generation includes:
- Text Planning
- Sentence Planning
- Text Realization
Q11. Explain Fuzzy Logic architecture.
- Fuzzification Module − The system inputs are fed into the Fuzzifier, which transforms the inputs into fuzzy sets.
- Knowledge Base − It stores analytic measures such as IF-THEN rules provided by experts.
- Inference Engine − It simulates the human reasoning process by making fuzzy inference on the inputs and IF-THEN rules.
- Defuzzification Module − It transforms the fuzzy set obtained by the inference engine into a crisp value.
Q12. Explain the components of Expert Systems.
- Knowledge Base
It contains domain-specific and high-quality knowledge. - Inference Engine
It acquires and manipulates the knowledge from the knowledge base to arrive at a particular solution. - User Interface
The user interface provides interaction between the user and the Expert System itself.
Q13. Which is better for image classification? Supervised or unsupervised classification? Justify.
- In supervised classification, the images are manually fed and interpreted by the Machine Learning expert to create feature classes.
- In unsupervised classification, the Machine Learning software creates feature classes based on image pixel values.
Therefore, it is better to choose supervised classification for image classification in terms of accuracy.
Q14. Finite difference filters in image processing are very susceptible to noise. To cope up with this, which method can you use so that there would be minimal distortions by noise?
Image Smoothing is one of the best methods used for reducing noise by forcing pixels to be more like their neighbors, this reduces any distortions caused by contrasts.
Q15. What is the Minimax Algorithm? Explain the terminologies involved in a Minimax problem.
Minimax is a recursive algorithm used to select an optimal move for a player assuming that the other player is also playing optimally.
A game can be defined as a search problem with the following components:
- Game Tree: A tree structure containing all the possible moves.
- Initial state: The initial position of the board and showing whose move it is.
- Successor function: It defines the possible legal moves a player can make.
- Terminal state: It is the position of the board when the game ends.
- Utility function: It is a function which assigns a numeric value for the outcome of a game.
Q16. How is Game theory and AI related?
“In the context of artificial intelligence(AI) and deep learning systems, game theory is essential to enable some of the key capabilities required in multi-agent environments in which different AI programs need to interact or compete in order to accomplish a goal.”
You May Also Like
These Related Stories
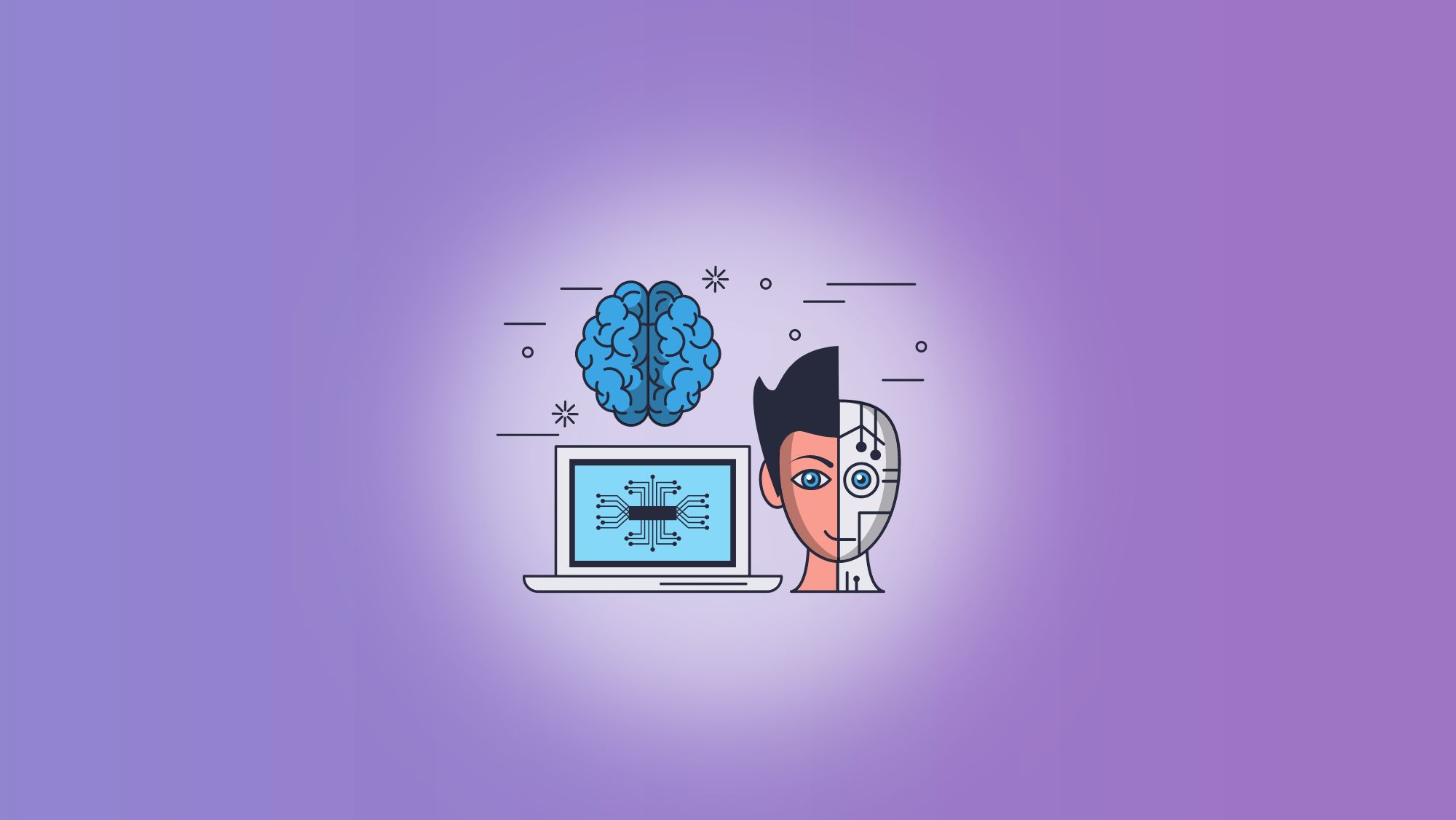
What is AI (Artificial Intelligence) ?
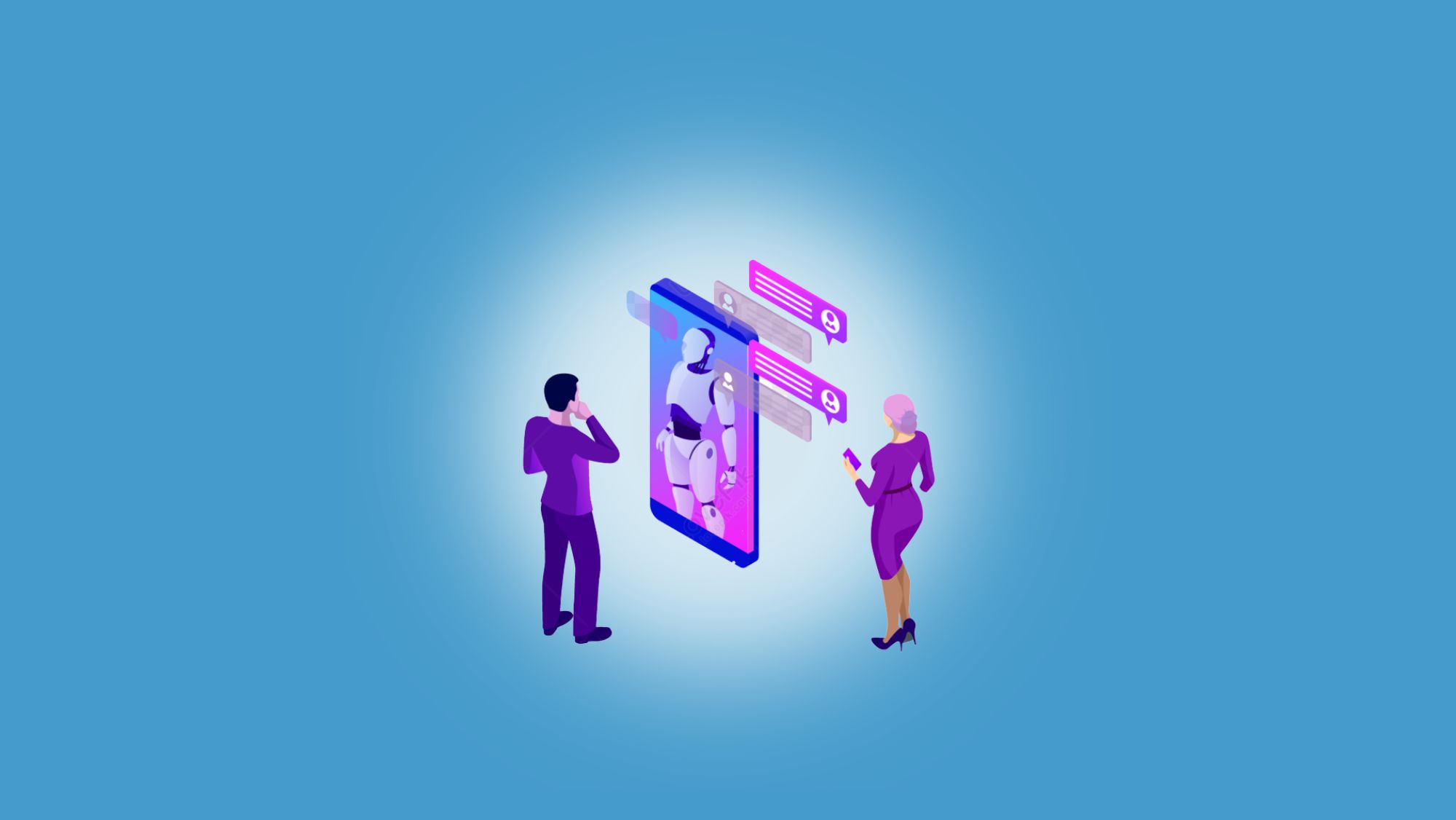
Is Artificial Intelligence Possible?
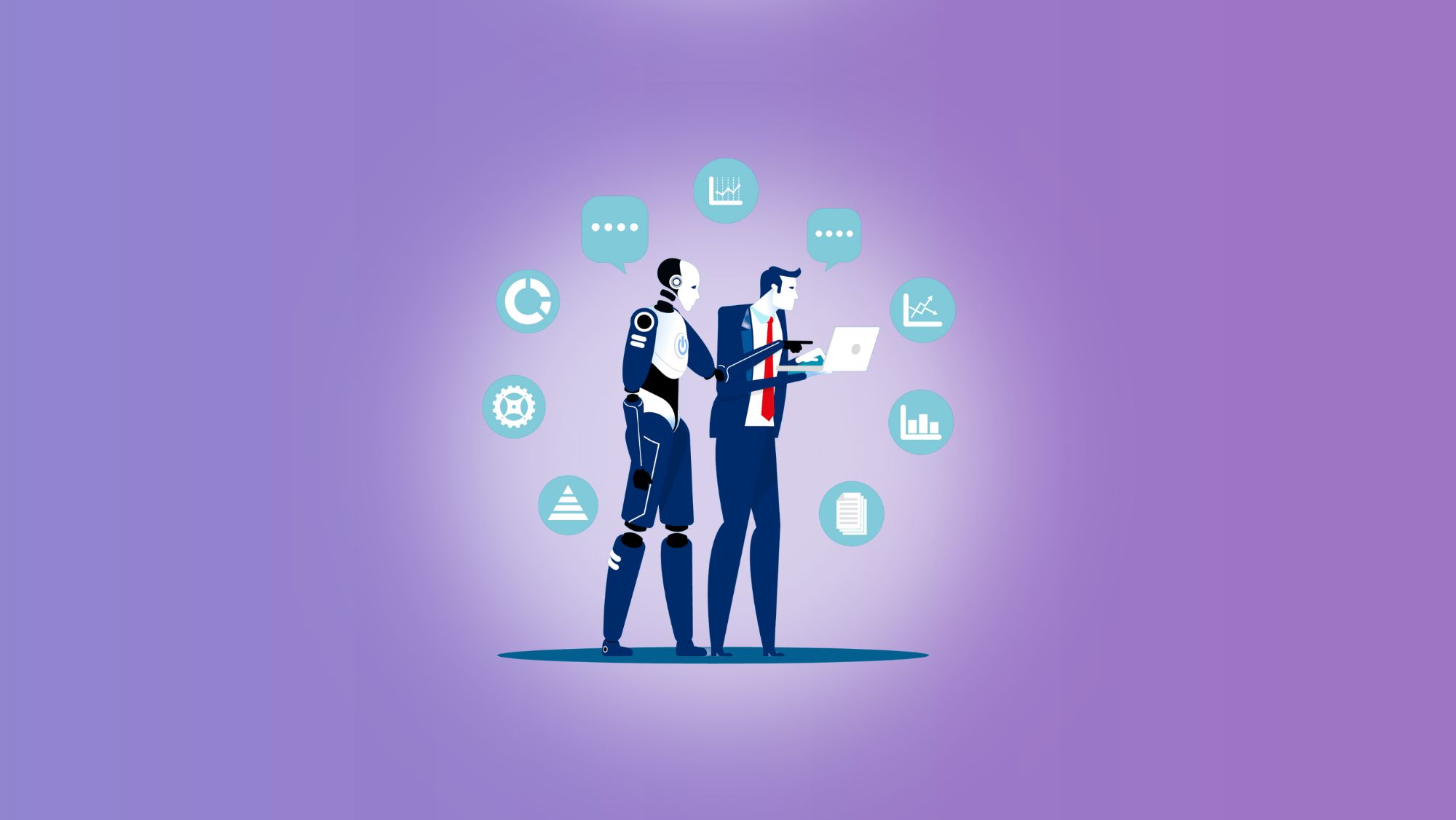
No Comments Yet
Let us know what you think